Point break: using machine learning to uncover a critical mass in women's representation
Source: Cambridge University Press
Decades of research has debated whether women first need to reach a “critical mass” in the legislature before they can effectively influence legislative outcomes. This study contributes to the debate using supervised tree-based machine learning to study the relationship between increasing variation in women's legislative representation and the allocation of government expenditures in three policy areas: education, healthcare, and defense. We find that women's representation predicts spending in all three areas. We also find evidence of critical mass effects as the relationships between women's representation and government spending are nonlinear. However, beyond critical mass, our research points to a potential critical mass interval or critical limit point in women's representation. We offer guidance on how these results can inform future research using standard parametric models.
Click here to read the full article published by Cambridge University Press on 21 September 2021.
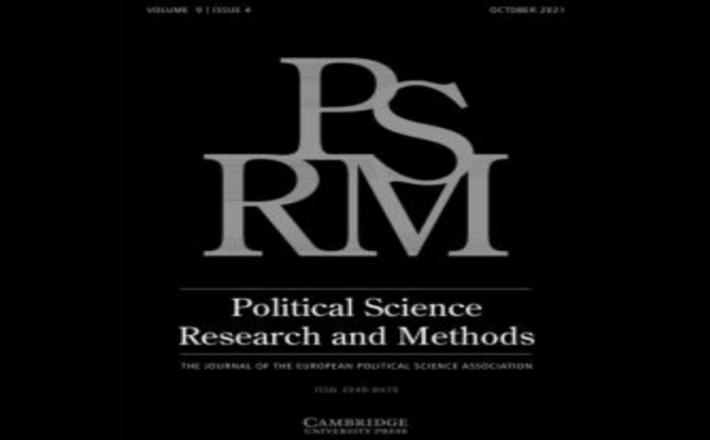
Decades of research has debated whether women first need to reach a “critical mass” in the legislature before they can effectively influence legislative outcomes. This study contributes to the debate using supervised tree-based machine learning to study the relationship between increasing variation in women's legislative representation and the allocation of government expenditures in three policy areas: education, healthcare, and defense. We find that women's representation predicts spending in all three areas. We also find evidence of critical mass effects as the relationships between women's representation and government spending are nonlinear. However, beyond critical mass, our research points to a potential critical mass interval or critical limit point in women's representation. We offer guidance on how these results can inform future research using standard parametric models.
Click here to read the full article published by Cambridge University Press on 21 September 2021.